Verticals
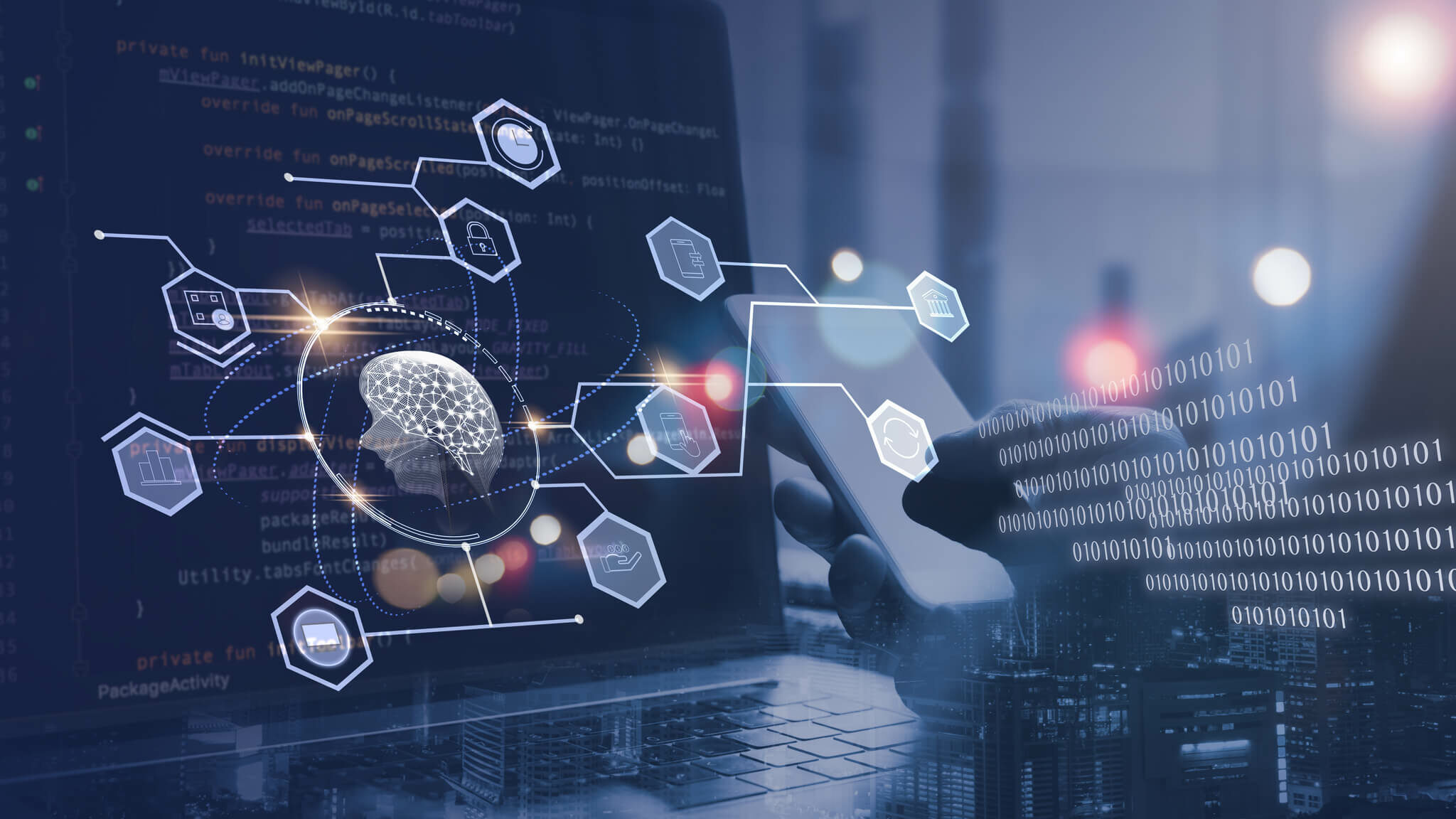
AI and Education
Artificial intelligence (AI) technologies can be used in a variety of ways in education, from providing personalised learning experiences to automating administrative tasks.
One of the most significant applications of AI in education is personalised learning. AI algorithms can analyse student data and adapt learning experiences to meet the needs and preferences of individual learners. This can include recommending personalised content, providing feedback and support, and tracking progress and performance.
AI can also be used to automate administrative tasks, such as grading assignments and providing feedback. This can save teachers significant time and effort, allowing them to focus more on teaching and student engagement.
Another potential application of AI in education is in the development of intelligent tutoring systems. These systems use AI algorithms to provide real-time feedback and guidance to students as they work through problems and exercises, improving student engagement and learning outcomes.
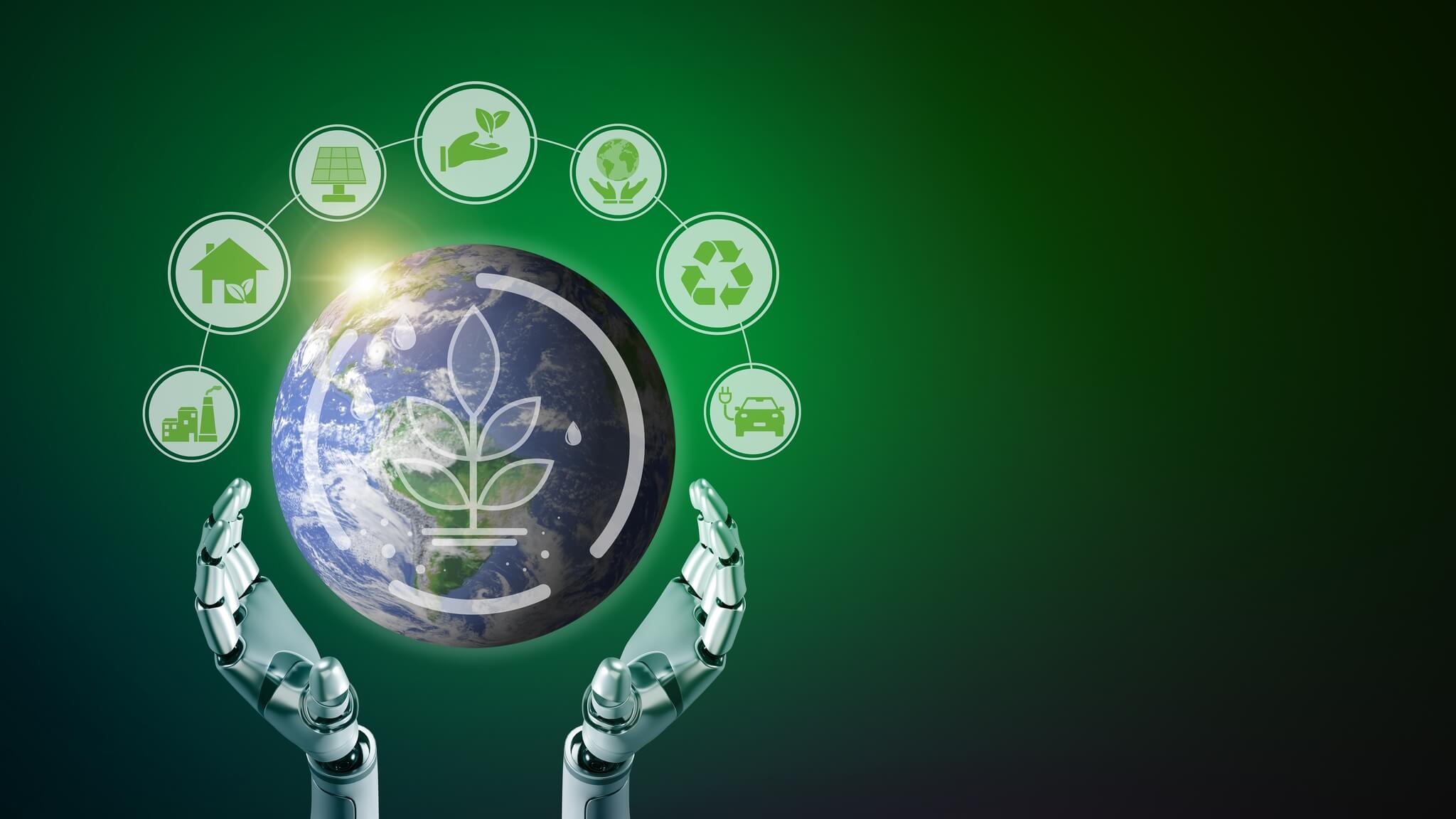
AI and Sustainability
AI and sustainability refer to the development and use of artificial intelligence (AI) technologies in a way that promotes long-term sustainability and mitigates their negative impacts on society and the environment. It involves considering the entire lifecycle of AI, from the design and development phase to the disposal or repurposing of the technology.
AI and Sustainability focused on developing AI systems that are not only effective and efficient but also ethical, transparent, and responsible. This means that AI technologies should be designed to respect human rights, privacy, and dignity, and should not discriminate against any group of people. AI and sustainability also involve designing systems that are transparent and explainable, so that users can understand how decisions are being made and identify any potential biases or errors.
In addition to ethical considerations, AI and Sustainability also focuses on minimising the environmental impact of AI technologies. This includes reducing the energy consumption and carbon footprint of AI systems, using sustainable materials in their production, and minimising waste and pollution throughout their lifecycle.
AI plays a crucial role in advancing sustainable energy systems by optimising operations, improving efficiency, and enabling better decision-making in various aspects of energy production, distribution, and consumption. The ability to process large amounts of data, make real-time decisions, and learn from complex systems enables the transition towards a sustainable and greener future.
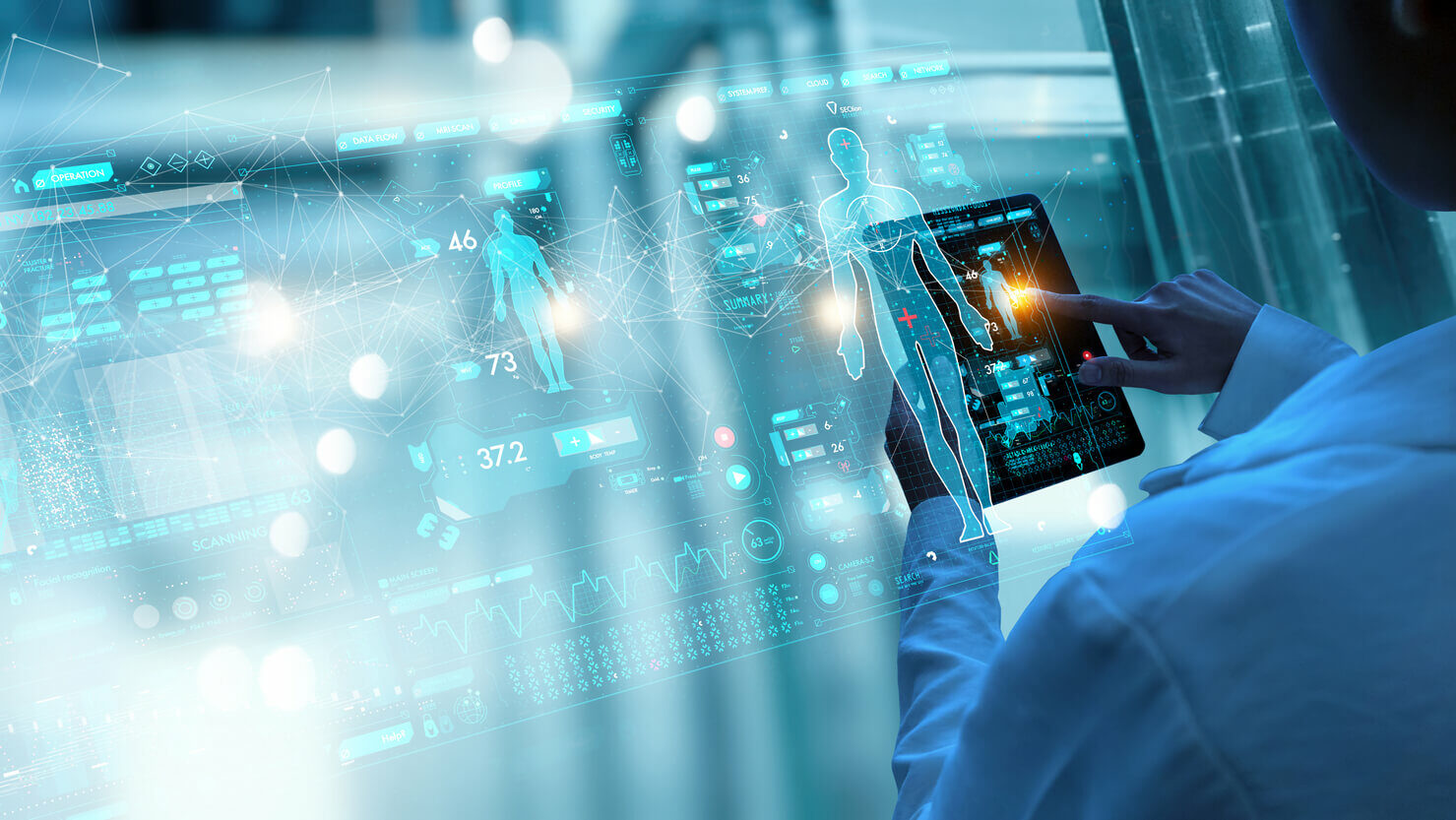
AI in Healthcare and Life Science
Artificial intelligence (AI) is transforming the healthcare and life science industries in numerous ways, offering solutions to long-standing problems, and bringing about innovations that were once unimaginable. From drug development to medical imaging analysis, AI is making a significant impact on how healthcare and life science are delivered.
One of the primary ways AI is transforming healthcare is by improving medical diagnosis and treatment. By analysing patient data, such as medical history, lab test results, and imaging scans, AI can help healthcare professionals make faster and more accurate diagnoses. AI algorithms can also develop personalised treatment plans based on a patient's unique characteristics, such as genetics, age, and lifestyle.
Electronic health records (EHRs) are another area where AI can improve the accuracy and efficiency of healthcare delivery. Natural language processing algorithms can extract important information from medical records and convert it into structured data, making it easier for healthcare professionals to access and use.
Overall, AI has the potential to revolutionise healthcare and life science industries by improving patient outcomes, reducing costs, and accelerating innovation. As AI technology continues to advance, it is likely to become an essential component of healthcare delivery and bring about significant improvements in the quality of care.
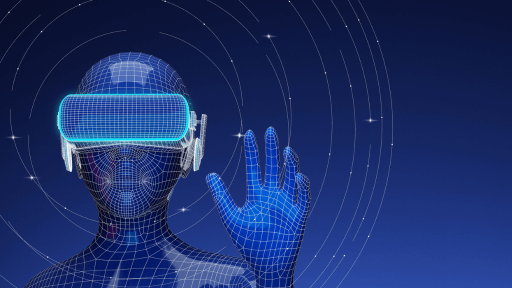
AI in Metaverse
The concept of the "metaverse" revolves around creating a collective virtual shared space, bridging the gap between the physical and virtual worlds using "XR" technology such as VR, AR, and MR.
The initiative "AI in Metaverse", under the broader "AI in XR" vertical, seeks to convene top-tier experts and academics to foster interdisciplinary collaboration li AR/VR, computer graphics, computer vision, robotics, and artificial intelligence. This will address the intricacies and challenges inherent in creating immersive educational simulations with the latest VR/AR/MR technologies.
Further discussions will penetrate deeper into the capabilities of contemporary extended reality (VR/AR/MR) for both enterprise and consumer applications, and the craft of producing immersive 3D virtual content that promises transformative societal impacts. IEEE CAI 2024 welcomes a spectrum of researchers and professionals from diverse computational domains related to XR technologies and content design for submissions of research papers that align with the following topics:
- Scene Understanding and Augmentation in XR
- AI-driven Interaction in XR
- Gesture recognition and interpretation
- Personalised content delivery in XR
- Procedural content generation for immersive environments
- Smart wearable devices and their integration into XR
- AI-powered scenario generation for training in XR
- AI-mediated interactions in social XR
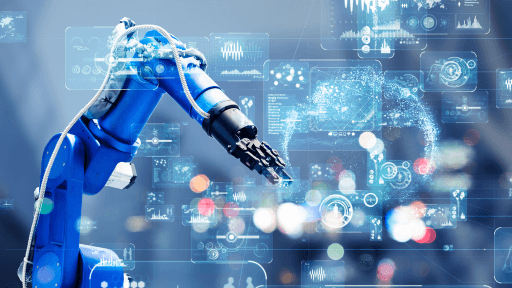
AI in Multi-agents and Robotic Systems
Multi-Agent Systems (MAS), as one of the very important subareas of Artificial Intelligence, is focused on the studies of how multiple intelligent agents or robotics cooperate, coordinate, and negotiate with each other, to accomplish delegated tasks. This includes two distinct scenarios where 1) agents or robotics share the same goal and 2) they have their own interests. Many useful systems have been developed in various application domains. The recent trend also goes beyond the scenario of pure agents or robotics and introduces an interesting and complex system of a mixture of agents and humans. This induces challenging research questions like how humans could trust the agents and robotics and how to ensure the humans would not be harmed by those agents and robotics in the system. Research contributions are solicited to tackle these problems.
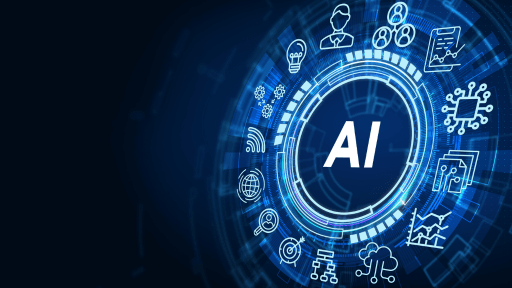
Foundation Models and Generative AI
Delve into the power of foundation models and generative AI, leveraging knowledge representation, crossing modality gaps, and reshaping data generation. It harnesses the potential of generative AI to innovate and ensure robustness, fairness, transparency, while also understanding the challenges involving model interpretability, knowledge representation, intelligent control, and social ethics in deploying the foundation models.
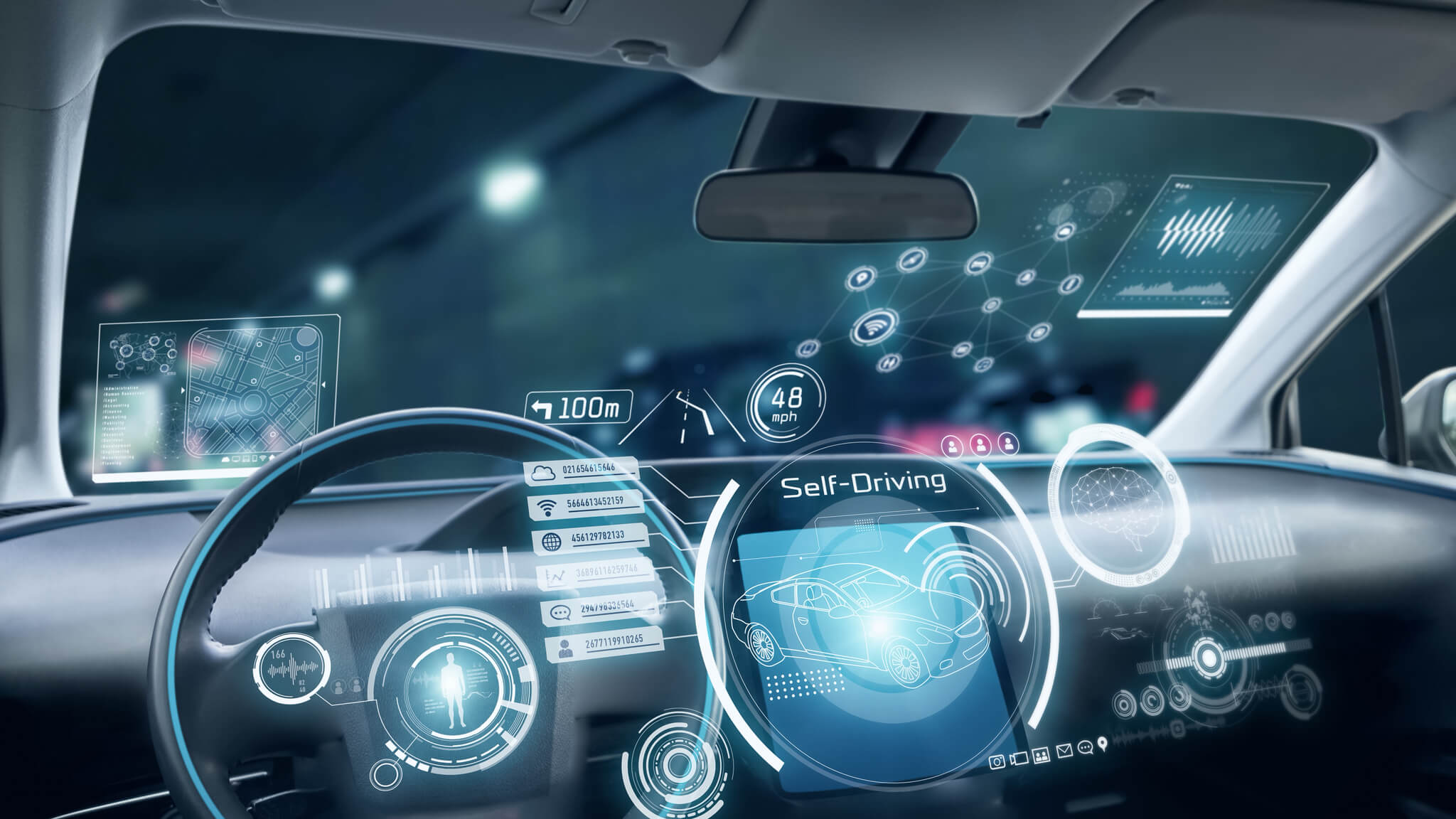
Industrial AI
Industrial AI, also known as AI in industry, refers to the application of artificial intelligence (AI) technologies to various industrial and manufacturing processes. Industrial AI is being used to improve efficiency, productivity, and quality in a wide range of industries, including automotive, aerospace, energy, healthcare, and many others.
In the aerospace industry, industrial AI is being used to improve aircraft design and maintenance, as well as air traffic management. AI algorithms can analyse data from sensors and other sources to identify potential issues with aircraft systems, allowing for proactive maintenance and reducing the risk of mechanical failures. AI can also be used to optimise air traffic flow, reducing delays, and improving safety.
In the transportation industry, industrial AI is being used to improve efficiency and safety in various modes of transportation, including cars, trucks, and trains. For example, self-driving cars rely on AI to navigate roads and make decisions in real-time. AI algorithms can also be used to optimise routes and reduce fuel consumption, improving both efficiency and sustainability.
In the maritime industry, industrial AI is being used to improve safety, efficiency, and environmental sustainability. AI can be used to optimise ship routing, reducing fuel consumption and emissions. AI algorithms can also be used to monitor ship systems and identify potential issues before they occur, improving safety and reducing downtime.
AI in manufacturing is used to enhance various aspects of the manufacturing process through the key elements such as Data Analysis, Predictive Maintenance, Quality Control, Process Optimisation, Energy Efficiency and Safety. This brings intelligence to the factory floor, making operations more efficient, accurate, and adaptable while improving quality, reducing costs, and increasing overall productivity.
In applications of AI to power systems, there are now various applications to power systems that enhance efficiency, reliability, and sustainability. This presents the potential to revolutionise power systems through real-time insights, predictive capabilities, and automation leading to efficient, reliable, and sustainable energy production, distribution, and consumption.
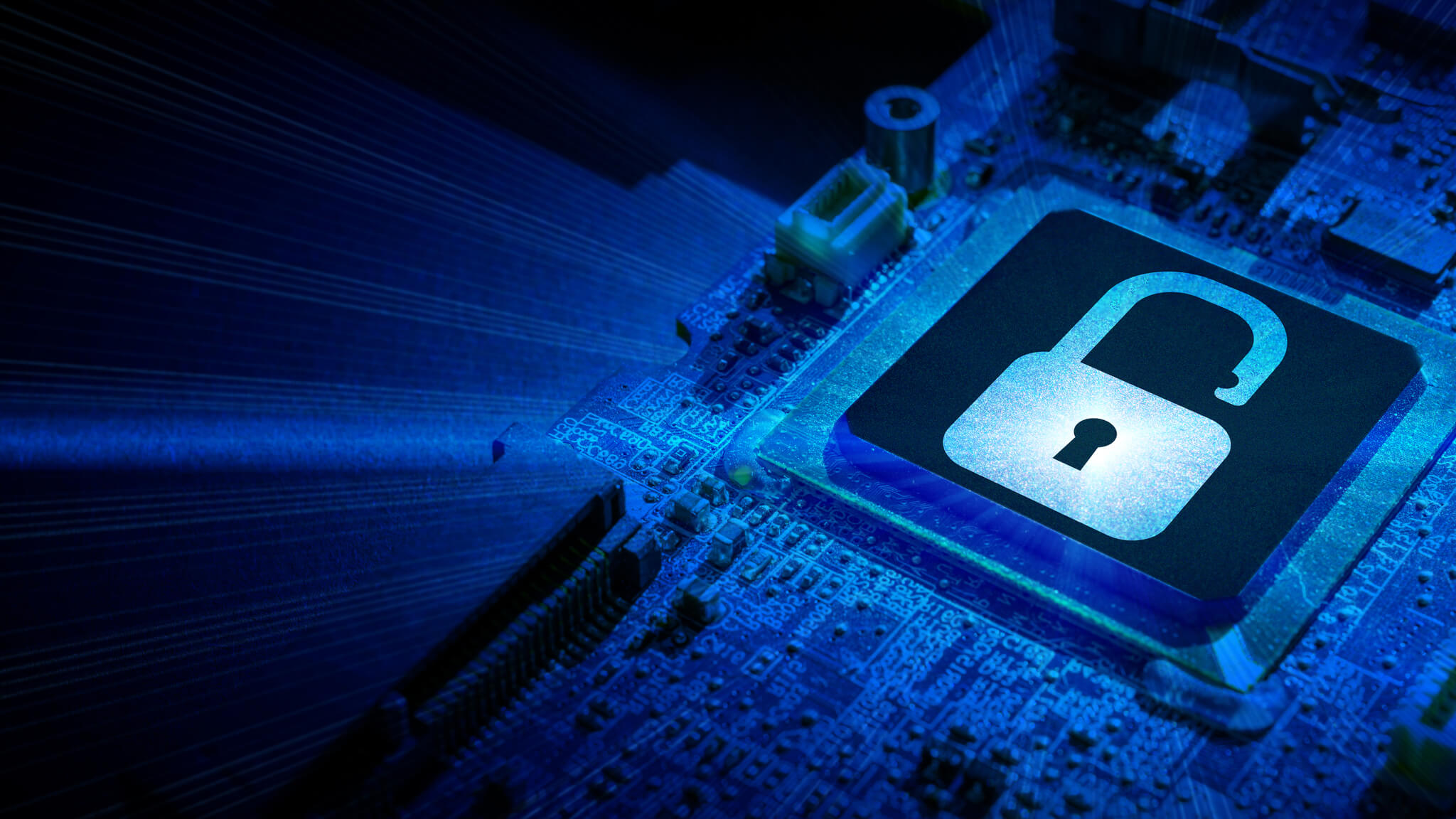
Resilient and Safe AI
Resilient and safe AI is increasingly important as artificial intelligence becomes more integrated into our daily lives. The use of AI in fields such as healthcare, finance, and transportation have the potential to improve efficiency and accuracy, but it also presents significant challenges in terms of reliability, security, and ethics. Resilient AI systems are essential for ensuring that AI can operate effectively and consistently, even in the face of unexpected events, while safe AI systems are critical for protecting the well-being of individuals and society as a whole.
In addition to resilience, safe AI systems must also address ethical and social concerns. One of the biggest concerns in AI development is the issue of bias. AI systems can be biased in various ways, including biased data, biased algorithms, and biased interpretations. These biases can have serious consequences, including discrimination, unfair treatment, and perpetuation of social inequalities.
Ensuring the safety of AI systems also requires addressing issues such as transparency and accountability. Users of AI systems must be able to understand how the system works and how it arrives at its decisions. This is particularly important in fields such as healthcare and finance, where decisions made by AI systems can have significant consequences for individuals and society as a whole.
Finally, safe AI systems must also address issues of data privacy and security. AI systems often rely on large amounts of data, much of which may be sensitive or personal in nature. Ensuring that this data is handled securely and ethically is critical for maintaining public trust in AI.
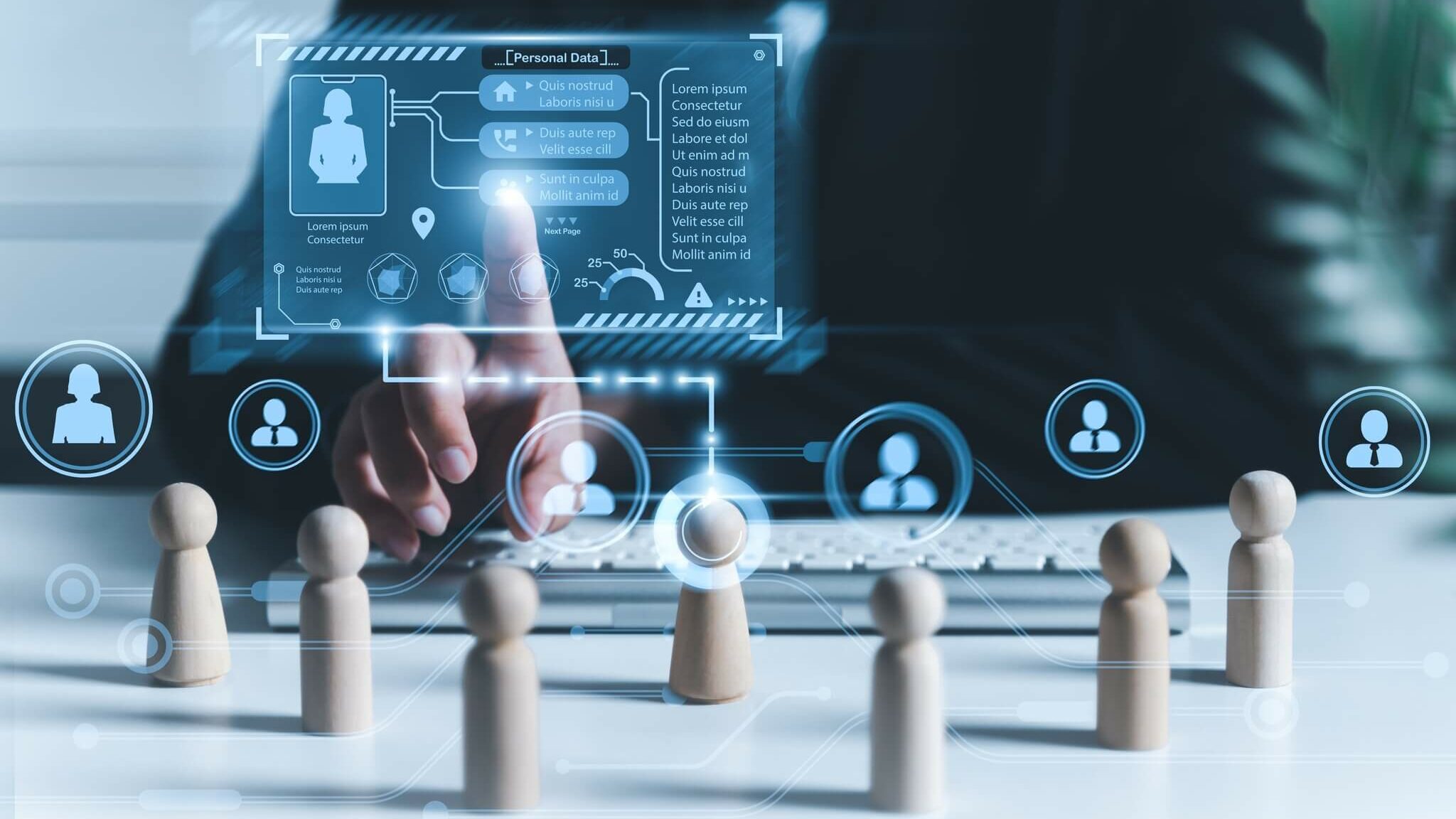
Societal Implications of AI
The societal implications of AI are complex and far-reaching, affecting everything from the job market to privacy and ethics. As AI technologies continue to advance, it is important that we address these issues proactively to mitigate any negative impacts. This requires a multidisciplinary approach that involves experts from fields such as technology, ethics, policy, and social sciences. Governments, businesses, and other organisations also have a critical role to play in ensuring that AI is developed and deployed in a responsible and ethical manner. By working together and prioritising transparency, fairness, and accountability, we can harness the potential of AI while minimising any adverse effects on society.
Governments, businesses, and other organisations also have a critical role to play in ensuring that AI is developed and deployed in a responsible and ethical manner. By working together and prioritising transparency, fairness, and accountability, we can harness the potential of AI while minimising any adverse effects on society.